Hilde G. Corneliussen, senior researcher and research director at the Western Norway Research Institute, has co-written the report "The use of artificial intelligence in the public sector and the risk of discrimination" with Aisha Iqbal, Gilda Seddighi and Rudolf Andersen.
"We start with the definition of artificial intelligence (AI) used in the national strategy and by the EU. The definition is very broad," says Corneliussen.
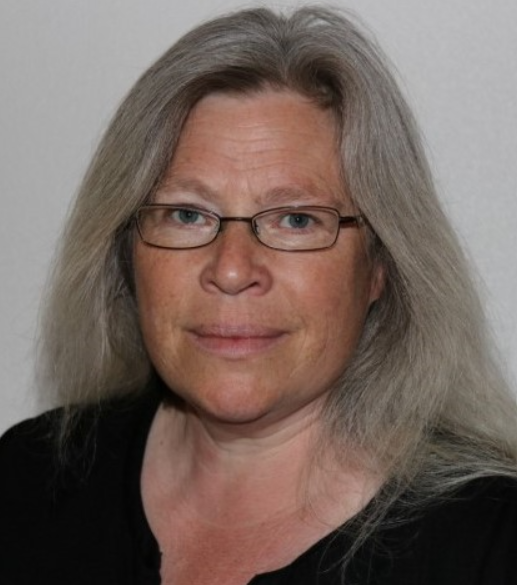
Artificial intelligence is defined as technologies that use algorithms to process data.
"This includes everything from the automation of simple procedures to very complex forms of machine learning," Corneliussen elaborates.
"In complex forms of machine learning, the processes take place in what are known as 'black boxes', meaning that the processes are so complicated that it’s difficult for humans to understand what is happening.”
According to Corneliussen, this makes AI both powerful and frightening, and it is therefore vital to be aware of the risk of discrimination when using AI in the public sector.
"Nevertheless, only three per cent of public institutions that participated in the survey believe that AI increases the risk of discrimination," she says.
The report "The use of artificial intelligence in the public sector and the risk of discrimination" was commissioned by Bufdir and prepared by the Western Norway Research Institute and the consultancy firm Ramboll.
The report was published in December 2022.
The purpose of the report is to map the use of artificial intelligence (AI) in the public sector that includes personal or individual data.
The report will provide a knowledge base for developing strategies to counteract the discriminatory effects of AI.
What is artificial intelligence?
"AI is a system of algorithms and data and is about recognising patterns in large amounts of data," Corneliussen explains.
“ChatGPT recently swept the world, but it would be wrong to label this type of artificial intelligences as ‘intelligent’. It's essentially about recognising patterns, both in the simpler versions of AI and in the more advanced ones," she says.
While algorithms are sets of instructions to produce an outcome, "machine learning" means that algorithms update themselves based on the outcomes they have produced. For example, a machine-learning algorithm could be one that learns to tell the difference between cats and dogs. Each time the algorithm is able to recognise a dog, it will update the "dog" category to improve its subsequent recognition of other dogs.
A simple automation procedure may be a chatbot that knows the right question to ask based on your response to the previous question.
"Machine learning relies on data to train the algorithm," Corneliussen explains.
"We are then talking about large amounts of data known as 'big data’.”
AI in its infancy in the public sector
The report Corneliussen has co-written is based on a survey and in-depth interviews with representatives from public institutions.
"We sent the survey to almost 500 institutions in both the municipal and state sectors," says Corneliussen.
"Two hundred institutions responded to us and 28 per cent of these had plans to start using AI or were working on projects that already use AI.
We were surprised that very few organisations had reached their goal of implementing AI that uses personal data.
Corneliussen says that the extent to which AI was used in the institutions varied greatly and that the use of AI that uses personal data is in its infancy in the public sector in Norway.
"While carrying out the survey, we were surprised that very few organisations had reached their goal of implementing AI that uses personal data. We thought we would uncover and discuss AI at an algorithm level, but the data did not lead us in that direction.”
"The use of AI to process personal data is in its infancy in the public sector," she says.
Accurate data is discriminatory data
In general, there is little awareness about the risk of discrimination when using AI.
"When we asked about discrimination in accordance with the Anti-Discrimination Act, we often received responses that used alternative words such as openness, fairness and justifiable. But replacing the word discrimination also makes one think differently," says Corneliussen.
"A number of institutions responded that discrimination may arise later in the development of the AI tools. When we asked about which grounds for discrimination might be relevant, most pointed to gender, age and ethnicity," she says.
According to Corneliussen, in order to counteract discrimination in AI there must be a focus on discrimination right from the very start of the process.
"Discrimination cannot be a consideration you simply add on at the end," she says.
She believes that the challenge with AI is that it relies on big data to train algorithms.
"The data used is collected from the world as we know it, both in the present but also in the past. Historical data will show, for example, that men are more often in managerial positions than women, and the algorithms may then favour men over women for such positions,” she says.
"Accurate data that reflects the reality of the world in which we live is discriminatory, because we inherit historical discrimination.”
Corneliussen points out that it is important to remember that artificial intelligence spans a broad field of technologies.
"It's easier to identify where discrimination is taking place when it's just a question of automating tasks. In complex AI systems, discrimination can occur inside the black box, making it more difficult to understand why it's happening.”
"This is particularly critical in the public sector, which must be able to justify decisions that are made," she adds.
Lack of diversity in technology development
Petter Bae Brandtzæg is a professor at the Department of Media and Communication at the University of Oslo. He points out that it is not only data that contains biases, but also digital technology.
"Minorities and women can be discriminated against by AI because much of the data that is out there reflects how white men view and interpret the world. For example, the majority of Wikipedia articles are written by white men," he says.
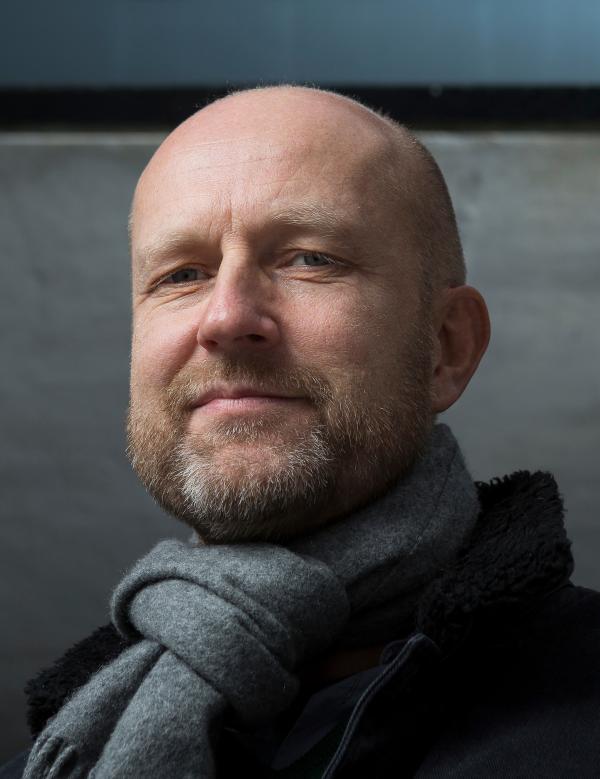
The problem is that AI models are often trained on existing data that does not reflect the diversity of society.
In addition, 90 per cent of people working in computer technology are men:
"It's an industry that lacks diversity, which means that digital technology is being designed by white men who see the world in a specific way.”
Nevertheless, it’s important to point out that society's institutions and elites in general also lack diversity:
"If we look at our ministries and other governing institutions, it’s clear that digital technology is not the only area of society that lacks diversity. There are several factors that may contribute to discrimination — not just AI,” he says.
"In the long term, perhaps even AI can help to strengthen diversity and reduce discrimination," he says.
Big data quality assurance
Like Corneliussen, Brandtzæg firmly believes that the quality assurance of data is essential to counteracting discrimination.
"When it comes to algorithms, it’s often said ‘bias in, bias out’. If you use data that does not take diversity into account when training algorithms, you also get discriminatory algorithms," he says.
Big data that is used to train algorithms is often taken from the internet, which is full of biases.
"In contrast, models can be trained that counteract discrimination and that contain human values so that the outcome is less discriminatory.”
“However, when it comes to tasks such as categorising groups, decisions or handling appeals, the processes must be monitored," says Brandtzæg.
"Big data that is used to train algorithms is often taken from the internet, which is full of biases," he says.
Thirty-nine of the two hundred institutions that responded to the survey had ongoing projects, or plans to use AI, that required personal data to train algorithms.
"It could be argued that the legislation is lagging a little behind. A public institution may be allowed to collect personal data for certain purposes, but not be allowed to use the same personal data to train algorithms," says Corneliussen.
She goes on to say that they have also uncovered issues related to expertise.
"Institutions in smaller municipalities may lack the in-house expertise to develop AI systems," she says.
"This means they have to rely on external expertise, which may make it more difficult to know on what data the technology is trained. It’s not certain that the data used to train the algorithms reflects the society that is using the technology," she says.
Need for increased awareness
Corneliussen points out the importance of working on the survey with an interdisciplinary perspective.
"We approached the topic from a sociological, technical and feminist perspective in order to understand the relationship between society, technology and discrimination," she says.
There is always a risk of discrimination when using AI, even if we do everything right.
According to the researcher, in order to combat discrimination, it is necessary that those developing AI tools are also onboard.
"There is always a risk of discrimination when using AI, even if we do everything right, but being aware of and combating discrimination should be included in all stages of the design and implementation of AI projects," she concludes.
A longer version of this article was first published in Norwegian